Open Access Article | Published in Algorithms
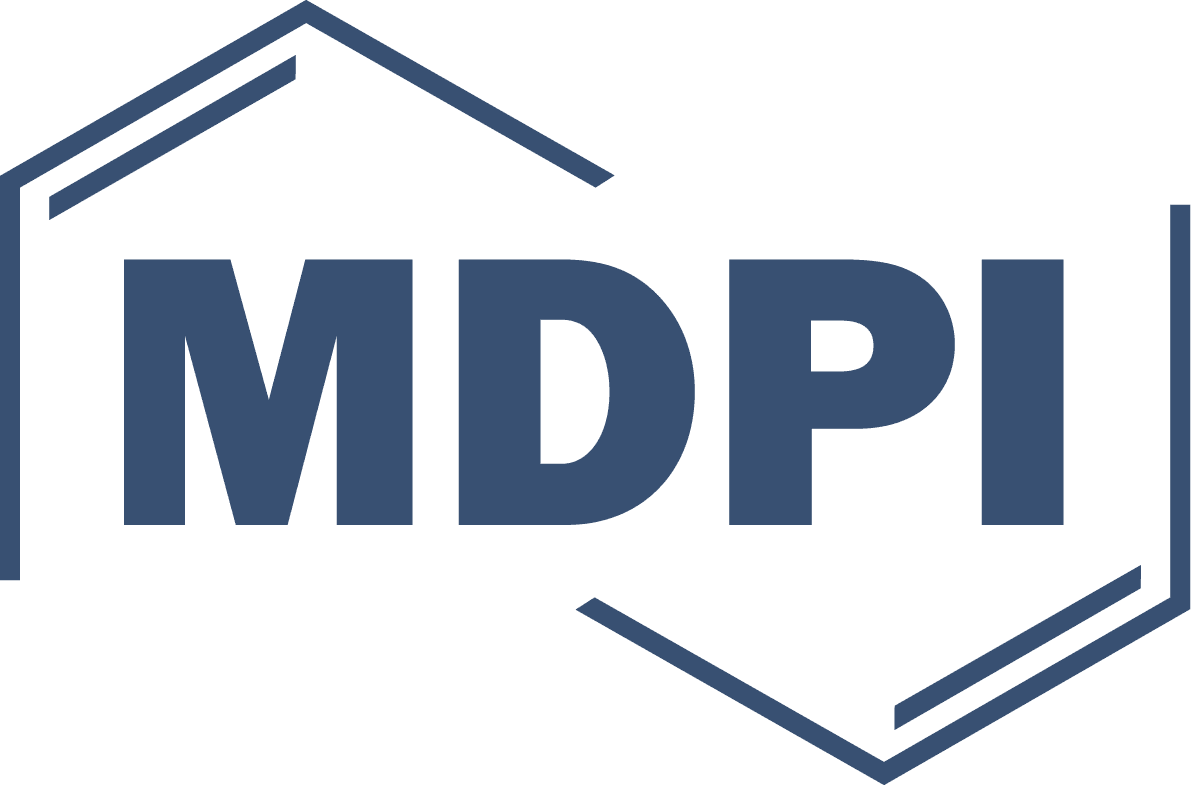
Abstract
In the last few years, fog computing has been recognized as a promising approach to support modern IoT applications based on microservices. The main characteristic of this application involve the presence of geographically distributed sensors or mobile end users acting as sources of data. Relying on a cloud computing approach may not represent the most suitable solution in these scenario due to the non-negligible latency between data sources and distant cloud data centers, which may represent an issue in cases involving real-time and latency-sensitive IoT applications. Placing certain tasks, such as preprocessing or data aggregation, in a layer of fog nodes close to sensors or end users may help to decrease the response time of IoT applications as well as the traffic towards the cloud data centers. However, the fog scenario is characterized by a much more complex and heterogeneous infrastructure compared to a cloud data center, where the computing nodes and the inter-node connecting are more homogeneous. As a consequence, the the problem of efficiently placing microservices over distributed fog nodes requires novel and efficient solutions. In this paper, we address this issue by proposing and comparing different heuristics for placing the application microservices over the nodes of a fog infrastructure. We test the performance of the proposed heuristics and their ability to minimize application response times and satisfy the Service Level Agreement across a wide set of operating conditions in order to understand which approach is performs the best depending on the IoT application scenario.
Link To original Paper
Related Projects
Artificial Intelligence Intern
AI Engineer intern, researching ways to adapt Visual Language Models for domain-specific data.
View ProjectCar Lo
I worked with two students on an IoT project using Arduino Uno, IMU, and GPS to improve driving styles and reduce CO2 emissions, with a demo app showing results.
View ProjectAws Solution Architect
The course introduced cloud computing, teaching me to deploy and manage open-source applications on AWS with a focus on cost constraints and Quality of Service (QoS).
View ProjectLocoBot
I fine-tuned Yolov5 on the Hagrid dataset to predict manual gestures for tracking and stopping, enhancing the robot's capabilities while retaining prior knowledge.
View ProjectMMR Driverless
As team leader, I coordinated the sensor system development for an autonomous vehicle, implemented the Stanley controller for trajectory tracking, and optimized skidpad performance for a formula student project.
View ProjectOvarian Cancer Survival Prediction
The project uses Graph Neural Networks on cancer patient data to enhance prediction precision by integrating multiple data sources with attention layers.
View ProjectArtificial Intelligence & Analytics Engineer
Full time artificial intelligence and analytics engineer consultant
View Project